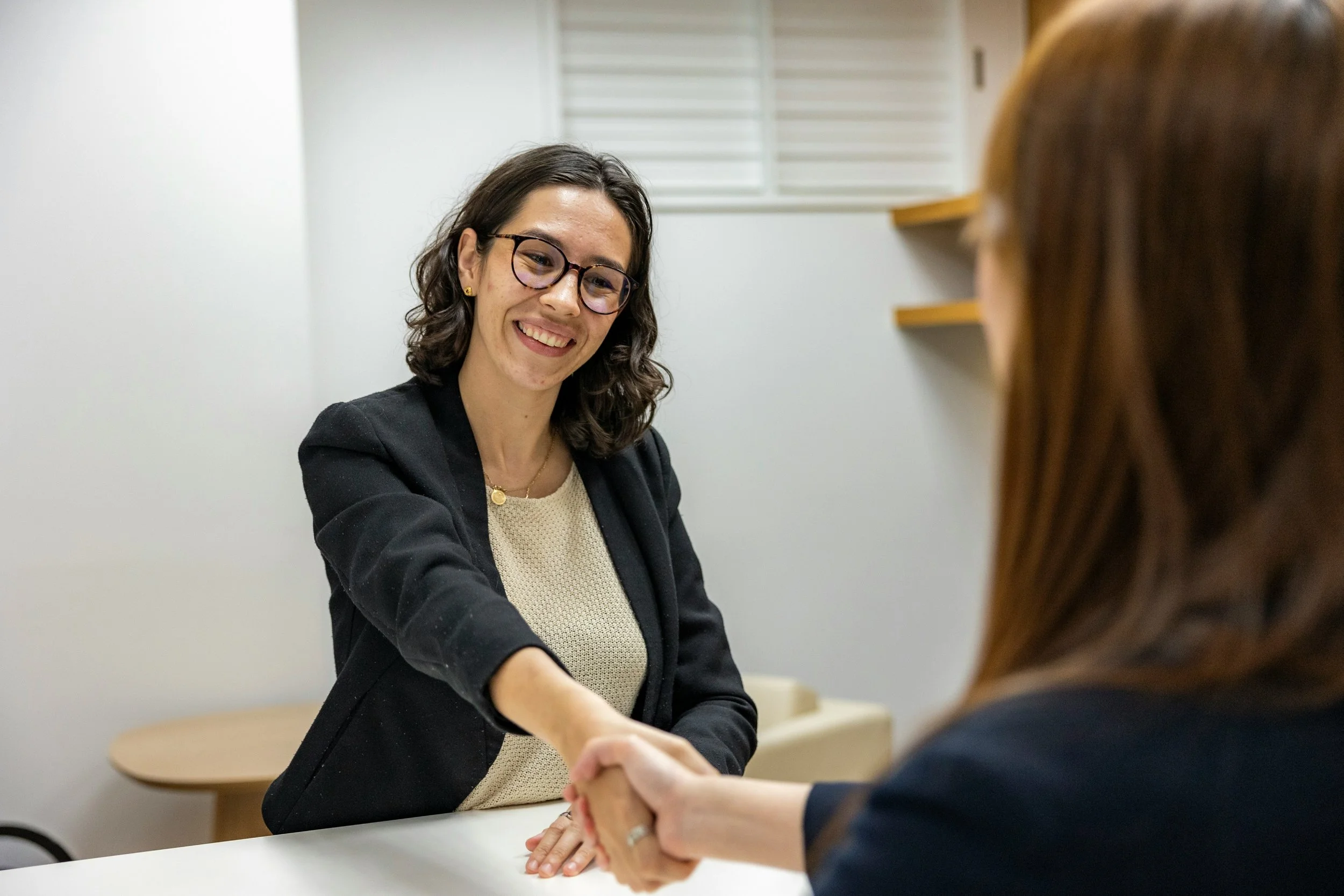
The fAIrbydesign story of HROS
What was the aim of the Use Case? Why should AI be used?
Which fairness risks were identified?
How could fairness risks be mitigated?
What did you do to mitigate fairness risks and what has changed as a result?
What has the UC partner learnt and taken away?
The aim of the Use Case:
The main aim of our system is to create a better, smoother experience for talents seeking and applying for jobs, by providing them with a matching platform with high quality recommendations for a next step in their careers.
The main benefit of our solution is that it saves job search time by doing “heavy lifting” in the background with our AI matching model. It allows users to focus on jobs that truly matter for their careers, improving the overall satisfaction of talents with our service.
The aim of the Fair by Design project:
The aim of the Fair by Design project is to ensure transparency and explainability of the HROS job matching solution. The project outline focuses on identification of strategies to mitigate risks associated with discrimination and bias as well as developing a toolbox - guidelines to proactively fight these identified problems.
As many AI systems are developed as powerful black boxes, the project aims to complement the technical side of development by considering implications on society and direct impact on users.
Purpose of using AI:
In our HR use case, the role of AI is to track thousands of career paths in our database to provide more informed job recommendations of the best next best job to talents. Our ML model is able to capture career progression as well as career transitions, providing users with more personalized insight about the next career possibilities.
What was the methodological approach?
Use of the AI model canvas and ecosystem analysis to build a good understanding of the use case and identify all relevant stakeholders, including possible affected groups
Mapping out the AI system and its functionalities and processes via the AI system mapping
Desk research to identify possible risks
Legal analysis
Technical analysis including code review and prediction system testing to identify bias and suitable mitigation strategies
Desk research and expert interviews to identify mitigation strategies
Use of Assurance Cases for fair AI systems to translate fairness claims into fairness requirements and evidences
Gender, seniority and location could influence the quality of matching
1. Continuous testing and monitoring of each system element and define thresholds for reevaluating and retraining.
3. Providing feedback options and transparency to inform about the matchings and detect any fairness risks.
2. Enabling human agency, explainability and useability for the users to be able to influence their career and make informed decisions.
4. The system consists of many parts, and uses some pre-defined AI components as well. Documentation of how the pieces fit together, the expected inputs and outputs for each component, would make it easier to track the impact of various sensitive features, and mitigate bias risks.
With the Assurance case, we were able to formulate fairness claims about our system and come up with specific evidences to back up those claims. For example, we claim that our system does not discriminate against certain groups of talents with further subclaim that our system does not discriminate against certain gender. With formulation of such statements, reasonings and assumptions, we were able to come up with specific testing methods. For instance, based on the assumption of gender bias, we came up with evidence to rule out gender bias in our system. We tested whether we can predict gender based on job history which would indicate gender bias.
For these, along with other types of systematic bias, we now have a foundation from which we can create more extensive tests and detection measures so that we can constantly evaluate the quality and performance of the model. These tests create a safety net, which can mitigate introducing new biases into the system resulting in the output that is considered unfair.
We identified different areas of our matching solution where bias may enter the process. Firstly by looking at the job matching system from a fairness perspective, we identified the limitations of our job recommendation system, as well as identified concrete steps to prevent biases entering the process.
We learnt that disregarding sensitive information from users does not automatically mean being unbiased or non-discriminatory.
We learnt that we can use descriptive user data (that may not necessarily be a part of training data) to test for biases and unfair treatment in the system. After the discovery of these biases we can then implement measures to remove them and test for them more thoroughly in future. This can lead to better model performance and a faster and safer development cycle of the model.
We learnt and formulated legal requirements of our high-risk AI system given the first draft of the new AI act published. Developing the legal side of the Assurance Case helped us to identify gaps when it comes to level of detail in our documentation, risk management system or transparency to the user.