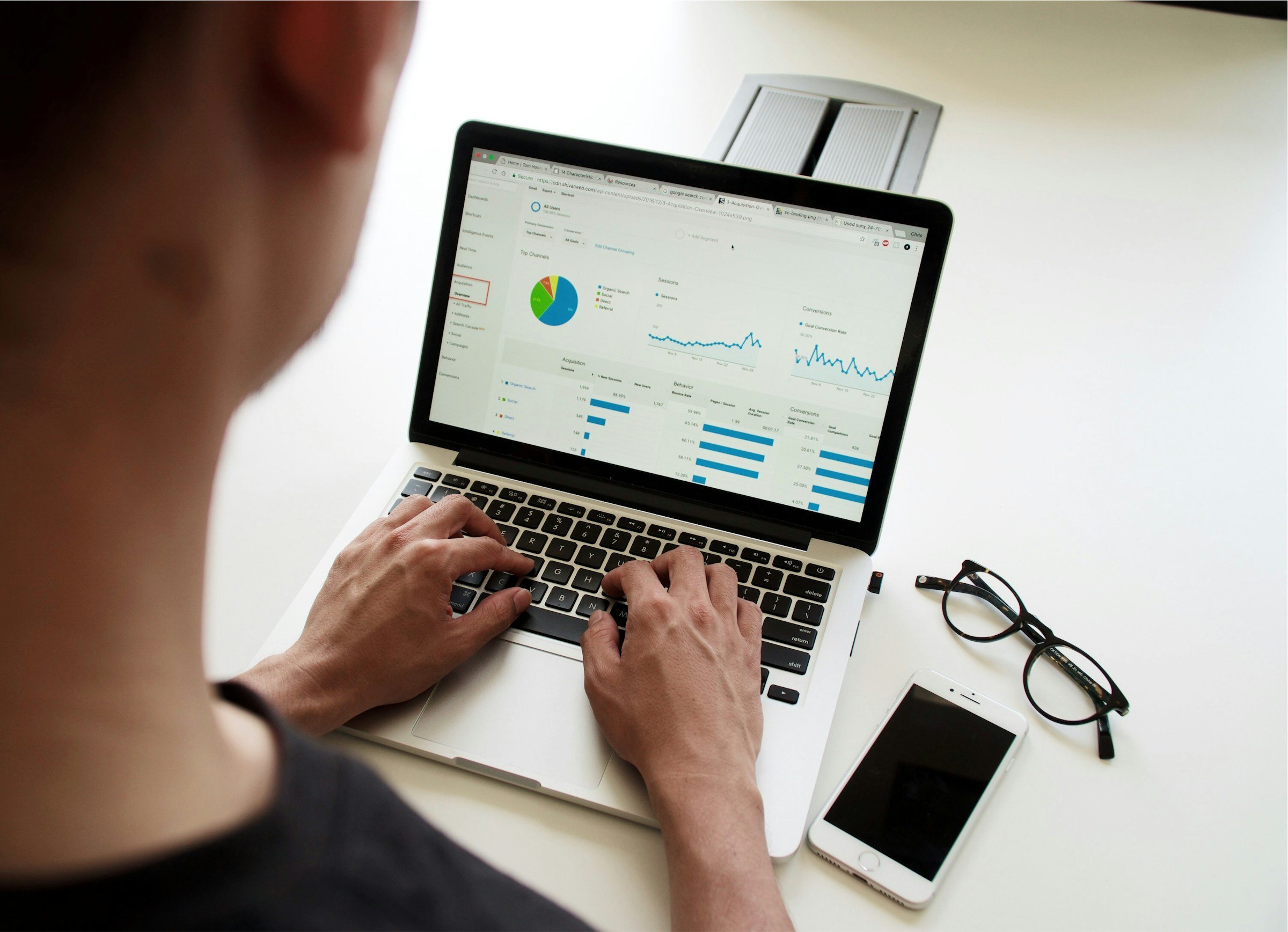
The fAIrbydesign story of Intact
What was the aim of the Use Case? Why should AI be used?
Which fairness risks were identified?
How could fairness risks be mitigated?
What did you do to mitigate fairness risks and what has changed as a result?
What has the UC partner learnt and taken away?
The UC of Intact in this project was an AI solution that supports audit reviewers in reviewing incoming audits. Since monitoring and checking those audits is a highly manual task, there is potential for partial automation of such checks. An AI assistant initially checks audits and guides the audit reviewer toward potential inconsistencies.
What was the methodological approach?
Use of the AI model canvas and ecosystem analysis to build a good understanding of the use case and identify all relevant stakeholders, including possible affected groups
Define the values of the company via the value sensitive design approach
Desk research to identify possible risks
Interviews with those potentially affected by the current system, to derive information on fairness requirements for an AI system
Legal analysis
Analysis of the findings, risk categorization
Prioritization of relevant fairness risks
Implementation of the algorithm based on open source code, in order to perform technical testing to detect fairness risks in the data and choice of algorithm, and to search for evidence of bias based on the risks identified in the desktop research and interviews.
Desk research and expert interviews, as well as technical analysis to identify mitigation strategies
Data that feeds into the system may be biased due to biased human assessments (for example certain individuals or groups may get disadvantageous treatment) or due to the assessment process that is used (may not be suitable for all kinds of inspected organizations)
The available data was insufficient for technical testing to ascertain risks for inspectors; however, there was, for example, some evidence to support bias risks for businesses that are new to being audited – a risk that was identified during the interview phase.
Technical tests revealed new risks, for example, associated with the type of food processing activity being pursued by the business, or related to the frequency of data aggregation for anomaly detection.
1. Design of the interface of the AI-system and training of the users to increase users’ understanding of the system outputs, and enable effective human agency and accountability;
3. Collection of further data to control for fairness: as described above, many risks could not be investigated due to lack of data. A targeted data collection and analysis plan would help verify if those risks apply.
2. Feedback loops to enable users, inspectors, and inspectees to report errors or inaccuracies
Internal awareness-raising of necessary considerations
Further data gathering and analysis of possible biases for UX and algorithms
Intact already developed its products according to relevant EU and international laws and guidelines. The research project pointed out necessary steps to fulfill the AI act that will be enforced soon
Fairness needs to be addressed already in the conceptual phase of product development
Furthermore, continuous monitoring and feedback gathering are needed to improve fairness parameters
User feedback is necessary as early as possible to consider different scenarios for UX and algorithm development