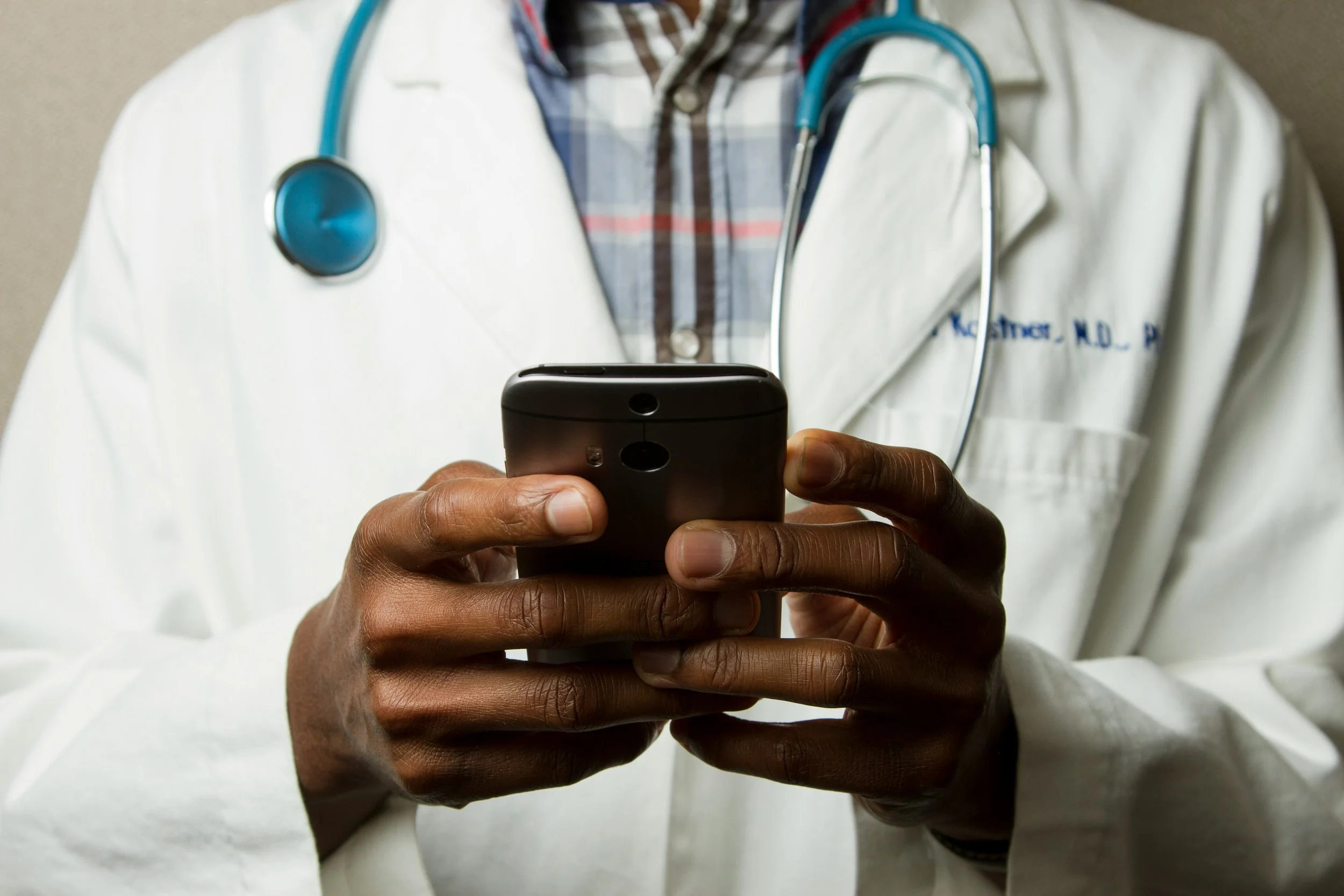
The fAIrbydesign story of rotable
What was the aim of the Use Case? Why should AI be used?
Which fairness risks were identified?
How could fairness risks be mitigated?
What did you do to mitigate fairness risks and what has changed as a result?
What has the UC partner learnt and taken away?
rotable is an early-stage tech start-up, that enables hospitals to simplify the clinical rotation scheduling of health care professionals through an innovative algorithm. The planning of medical rotations is highly complex and takes up valuable time of doctors. Thus, by using our algorithm we allow them to minimize time spent on admin tasks and increase the quality of rotation schedules.
Within the course of the project, different aspects and methods to develop the rotable algorithm were conceptualized, tested and evaluated. Therefore, the aim of the UC of rotable was to identify and test possible bias and fairness aspects that (could) arise in the first phases of the CDM process of a research project.
Find out more about the Use Case at: www.rotable.de
What was the methodological approach?
Use of the AI model canvas and ecosystem analysis to build a good understanding of the use case and identify all relevant stakeholders, including possible affected groups
Define the values of the company via the value sensitive design approach
Desk research to identify possible risks
Interviews with those potentially affected by the current system, to derive information on fairness requirements for an AI system
Legal analysis
Analysis of the findings, risk categorization
Prioritization of relevant fairness risks
Technical analysis
Desk research and expert interviews, as well as technical analysis to identify mitigation strategies
Use of Assurance Cases for fair AI systems to translate fairness claims into fairness requirements and evidences
Gender and origin (in particular, in relation to non-native German speakers) could be fairness risk factors
There is a need to differentiate between group-level fairness risks, and individual-level fairness risks, and this has implications for both the code implementation, and the types of tests that need to be run
For users of the system, a lack of transparency, useability, explainability and human agency were defined as fairness risks
For the AI subjects, a lack of transparency could result in undetected fairness risks
1. Careful documentation of optimization constraints, the assumptions behind them, as well as the choice of calculation methods for various variables, can help to identify possible biases and enables more effective testing.
3. Recording additional data only for fairness testing purposes and continuous monitoring of predefined fairness metrics and thresholds
2. Anomaly detection and feedback options to enable the detection of unknown fairness risks
3. Focusing on a high level of transparency and explainability for the users as well as the AI subjects
track and record data, that is not needed to create rotation schedules, but give us the option to test against fairness, discrimination and bias
Inform the users about predefined quality/fairness KPIs of a rotation schedule that serve as a basis for their decision making
implement multiple (additional) options for the users and the planned persons to give feedback on the automatically created schedules
make the automated scheduling process and underlying data as transparent as possible for both the planners and the planned persons
Fairness and bias aspects are prevalent in every project, but especially in ones where persons are planned. Hence, when using AI, it is highly valuable and important to take those aspects into account from day one. Only then awareness on (potential) issues can be anticipated and mitigation strategies can be created early on.
Additionally, it is not only important to track and record data that is needed to fulfil the purpose of your product, but rather one needs to makes sure, that the right data is recorded to then being able to check for bias and fairness aspects.
Having a clear and structured overview of your product – e.g. by using the Assurance Case – and of where and when bias/discrimination might arise, is crucial in order to take the right steps and to apply the right mitigation strategies. This is an ongoing process that takes time and needs to be updated constantly.